Primary Screening System for Sarcopenia in Elderly People Based on Artificial Intelligence
DOI:
https://doi.org/10.17488/RMIB.44.4.4Keywords:
anthropometric measure, elderly people, hierarchical clustering, Random Forest, sarcopeniaAbstract
This study proposes a primary screening system for diagnosing sarcopenia in older adults through anthropometric measures. This exploratory research initially involved 150 elderly individuals, of whom 122 were selected after a data purification process. Using machine learning techniques such as hierarchical clustering and decision trees, the original set of 13 anthropometric measures was reduced to five key features. Three classification systems were created: the first based on previously established parameters (appendicular muscle mass, walking speed, and grip strength); the second considered upper limb measures (average muscle mass of both arms, grip strength, walking speed, and body fat percentage); and the third focused on lower limb measures (average muscle mass of both legs, grip strength, walking speed, and body fat percentage). These classification systems were clinically validated in a group of 57 patients previously diagnosed by specialists, of which 10 received a positive sarcopenia diagnosis. The results showed similar efficiencies in all three systems, with eight of the ten known positive diagnoses classified in the same group. Additionally, the study provides specific cut-off points for each system, thus facilitating the clinical diagnosis of sarcopenia by medical professionals.
Downloads
References
B. Ballina Negrete, “La construcción institucional de la educación inclusiva en México,” Tesis de pregrado, Univ. Nac. Aut. Mex., Ciudad de México, México, 2023. [En línea]. Disponible en: https://ru.dgb.unam.mx/bitstream/20.500.14330/TES01000842167/3/0842167.pdf
L. F. Faúndez-Pino, “Envejecimiento saludable y enfoque salutogénico,” Rev. Mex. Med. Fam., vol. 10, no. 1, pp. 39-45, 2023. [En línea]. Disponible en: https://www.scielo.org.mx/scielo.php?script=sci_arttext&pid=S2696-12962023000100039
M. M. Hechavarría Ávila, M. Ramírez Romaguera, H. García Hechavarria y A. García Hechavarria, “El envejecimiento. Repercusión social e individual,” Rev. Inf. Cient., vol. 97, no. 6, pp. 1173-1188, 2018. [En línea]. Disponible en: http://scielo.sld.cu/scielo.php?pid=S1028-99332018000601173&script=sci_arttext
M. Casati, A. S. Costa, D. Capitanio, L. Ponzoni, E. Ferri, S. Agostini y E. Lori, “The Biological Foundations of Sarcopenia: Established and Promising Markers,” Front. Med., vol. 6, art. no. 184, 2019, doi: http://dx.doi.org/10.3389/fmed.2019.00184
H. Simsek, R. Meseri, S. Sahin, A. Kilavuz, et al., “Prevalence of sarcopenia and related factors in community-dwelling elderly individuals,” Saudi Med. J., vol. 40, no. 6, pp. 568–574, 2019, doi: https://doi.org/10.15537%2Fsmj.2019.6.23917
P. B. V. Fernández, A. E. Domínguez, I. B. Vázquez, C. A. Acosta, S. C. Diaz, D. D. A. Navarro, “Sarcopenia and related factors in women over 40 years,” Rev. Cuba. Endoc., vol. 31 no. 3, art. no. e198, 2020. [En línea]. Disponible en: https://www.medigraphic.com/cgi-bin/new/resumenI.cgi?IDARTICULO=100636
A. Casirati, G. Vandoni, S. Della Valle, G. Greco, M. Platania, S. Colatrugio, L. Lalli, C. Gavazzi, “Nutritional status and body composition assessment in patients with a new diagnosis of advanced solid tumour: Exploratory comparison of computed tomography and bioelectrical impedance analysis,” Clin. Nutr., vol. 40, no. 3, pp. 1268-1273, 2021, doi: http://dx.doi.org/10.1016/j.clnu.2020.08.009
W. A. Sepulveda Loyola, G. A. Luna Corrales, F. Ganz, H. Gonzalez Caro y V. Suziane Probst, “Sarcopenia, definición y diagnóstico: ¿Necesitamos valores de referencia para adultos mayores de Latinoamérica?,” Rev. Chil. Ter. Ocup., vol. 20, no. 2, pp. 259–267, 2020, doi: https://doi.org/10.5354/0719-5346.2020.53583
T. M. Nicolalde Cifuentes y S. I. Heredia Aguirre, “Parámetros antropométricos y su relación con la actividad física en una población adulta,” Polo Conoc., vol. 6 no. 9, pp. 723-736, 2021. [En línea]. Disponible en: https://dialnet.unirioja.es/servlet/articulo?codigo=8094563
K. Keller, “Sarcopenia,” Wien. Med. Wochenschr., vol. 169, pp. 157-172, 2019, doi: https://doi.org/10.1007/s10354-018-0618-2
M. C. Espinel-Bermúdez, S. Sánchez-García, C. García-Peña, X. Trujillo, M. Huerta-Viera, V. Granados-García, S. Hernández-González, y E. D. Arias-Merino, “Factores asociados a sarcopenia en adultos mayores mexicanos: Encuesta Nacional de Salud y Nutrición 2012,” Rev. Med. Inst. Mex. Seguro Soc., vol. 56, no. 1, pp. 46-53, 2018. [En línea]. Disponible en: https://www.redalyc.org/articulo.oa?id=457754907008
G. S. Collins y K. G. Moons, “Reporting of artificial intelligence prediction models,” The Lancet, vol. 393, no. 10181, pp. 1577-1579, 2019, doi: https://doi.org/10.1016/s0140-6736(19)30037-6
M. Banerjee, E. Reynolds, H. B. Andersson y B. K. Nallamothu, “Tree-based analysis: a practical approach to create clinical decision-making tools,” Cir. Cardiovasc. Qual. Outcomes, vol. 12, no. 5, art. no. e004879, 2019, doi: https://doi.org/10.1161%2FCIRCOUTCOMES.118.004879
S. O. Lugo-Reyes, G. Maldonado-Colín y C. Murata, “Inteligencia artificial para asistir el diagnóstico clínico en medicina,” Rev. Alerg. Mex., vol. 61, no. 2, pp. 110-120, 2014, doi: https://doi.org/10.29262/ram.v61i2.33
L. Breiman, “Random forests,” Mach. Learn., vol. 45, pp. 5-32, 2001, doi: https://doi.org/10.1023/A:1010933404324
G. T. Reddy, M. P. K. Reddy, K. Lakshmanna, R. Kaluri, D. S. Rajput, G. Srivastava y T. Baker, “Analysis of dimensionality reduction techniques on big data,” IEEE Access, vol. 8, pp. 54776-54788, 2020, doi: https://doi.org/10.1109/access.2020.2980942
R. Krzymińska-Siemaszko, E. Deskur-Śmielecka, A. Kaluźniak-Szymanowska, A. Styszyński y K. Wieczorowska-Tobis, “Polish version of SARC-F to assess sarcopenia in older adults: An examination of reliability and validity,” PLoS One, vol. 15, no. 12, art. no. e0244001, 2020, doi: http://dx.doi.org/10.1371/journal.pone.0244001
S. Huang, J. Yang, S. Fong y Q. Zhao, “Artificial intelligence in cancer diagnosis and prognosis: Opportunities and challenges,” Cancer Lett., vol. 471, pp. 61-71, 2020, doi: http://dx.doi.org/10.1016/j.canlet.2019.12.007
F. Morandín-Ahuerma, “Diez recomendaciones de la Unesco sobre ética de la inteligencia artificial,” En: Principios normativos para una ética de la inteligencia artificial. Puebla, México: Consejo de Ciencia y Tecnología del Estado de Puebla (CONCYTEP). pp. 86-94.
Cámara de Diputados del H. Congreso de la Unión. (7 de febrero de 1984). Ley General de Salud. [En línea]. Disponible en: https://www.diputados.gob.mx/LeyesBiblio/pdf/LGS.pdf
J. W. Osborne, Best practices in data cleaning: A complete guide to everything you need to do before and after collecting your data, Thousand Oaks, CA: Sage Publications Inc., 2013, doi: https://doi.org/10.4135/9781452269948
J. F. Ávila-Tomás, M. A. Mayer-Pujadas y V. J. Quesada-Varela, “La inteligencia artificial y sus aplicaciones en medicina II: importancia actual y aplicaciones prácticas,” Aten. Primaria, vol. 53, no. 1, pp. 81-88, 2020, doi: https://doi.org/10.1016/j.aprim.2020.04.014
S. H. Park y K. Han, “Methodologic guide for evaluating clinical performance and effect of artificial intelligence technology for medical diagnosis and prediction,” Radiology, vol. 286, no. 3, pp. 800-809, 2018, doi: http://dx.doi.org/10.1148/radiol.2017171920
L. Lera, C. Albala, H. Sánchez, B. Angel, M. J. Hormazabal, C. Márquez y P. Arroyo, “Prevalence of Sarcopenia in Community-Dwelling Chilean Elders According to an Adapted Version of the European Working Group on Sarcopenia in Older People (EWGSOP) Criteria,” J. Frailty Aging., vol. 6, no. 1, pp. 12-17, 2017, doi: https://doi.org/10.14283/jfa.2016.117
J. Moncada Jiménez, Estadística para ciencias del movimiento humano. Costa Rica: Editorial de la Universidad de Costa Rica, 2005.
S. Huang, J. Yang, S. Fong y Q. Zhao, “Artificial intelligence in cancer diagnosis and prognosis: Opportunities and challenges,” Cancer Lett., vol. 471, pp. 61-71, 2020, doi: http://dx.doi.org/10.1016/j.canlet.2019.12.007
A. T. Azar y S. M. El-Metwally, “Decision tree classifiers for automated medical diagnosis,” Neural Comput. Applic., vol. 23, pp. 2387–2403, 2013, doi: http://dx.doi.org/10.1007/s00521-012-1196-7
D. Blázquez García, “Análisis de datos de expresión en retina para la identificación de biomarcadores de DMAE: posible herramienta diagnóstica,” Tesis de pregrado, Fac. Med., Univ. Vall., Valladolid, España, 2023. [En línea]. Disponible en: https://uvadoc.uva.es/handle/10324/61377
F. T. T. Lai, P. E. Beeler, B. H. K. Yip, M. Cheetham, et al., “Comparing multimorbidity patterns among discharged middle-aged and older inpatients between Hong Kong and Zurich: A hierarchical agglomerative clustering analysis of routine hospital records,” Front. Med., vol. 8, art. no. 651925, 2021. https://doi.org/10.3389/fmed.2021.651925
P. Lemenkova, “R Libraries {dendextend} and {magrittr} and Clustering Package scipy.cluster of Python For Modelling Diagrams of Dendrogram Trees,” Carpathian J. Electr. Comp. Eng., vol. 13, no. 1, pp. 5-12, 2020, doi: https://doi.org/10.2478/cjece-2020-0002
F. Pedregosa, G. Varoquaux, A. Gramfort, V. Michel, et al., “Scikit-learn: Machine learning in Python,” J. Mach. Learn. Res., vol. 12, no. 85, pp. 2825−2830, 2011. [En línea]. Disponible en: http://jmlr.org/papers/v12/pedregosa11a.html
A. K. Castañeda Escobar, “Diseño e implementación de un algoritmo para determinar la mejor agrupación utilizando k-means y los índices de validación,” Tesis de maestría, Univ. Aut. Edo. Mor., Morelos, México, 2023. [En línea]. Disponible en: http://riaa.uaem.mx/handle/20.500.12055/3115
E. Goffinet, M. Lebbah, H. Azzag, L. Giraldi y A. Coutant, “Multivariate time series multi-coclustering: Application to advanced driving assistance system validation,” in Europ. Symp. Artif. Neural Netw. Comput. Intell. Mach. Learn., Oct. 2021, pp. 463-468, doi: https://doi.org/10.14428/esann/2021.es2021-119
S. J. Rigatti, “Random forest,” J. Insur. Med., vol. 47, no. 1, pp. 31-39, 2017, doi: http://dx.doi.org/10.17849/insm-47-01-31-39.1
M. Bakator y D. Radosav, “Deep Learning and Medical Diagnosis: A Review of Literature,” Multimodal Technol. Interact., vol. 2, no. 3, art. no. 47, 2018, doi: https://doi.org/10.3390/mti2030047
A. Yuki, F. Ando, R. Otsuka, Y Matsui, A. Harada, H. Shimokata, “Epidemiology of sarcopenia in elderly Japanese,” J. Phys. Fitness Sports Med., vol. 4, no. 1, pp. 111-115, 2015, doi: http://dx.doi.org/10.7600/jpfsm.4.111
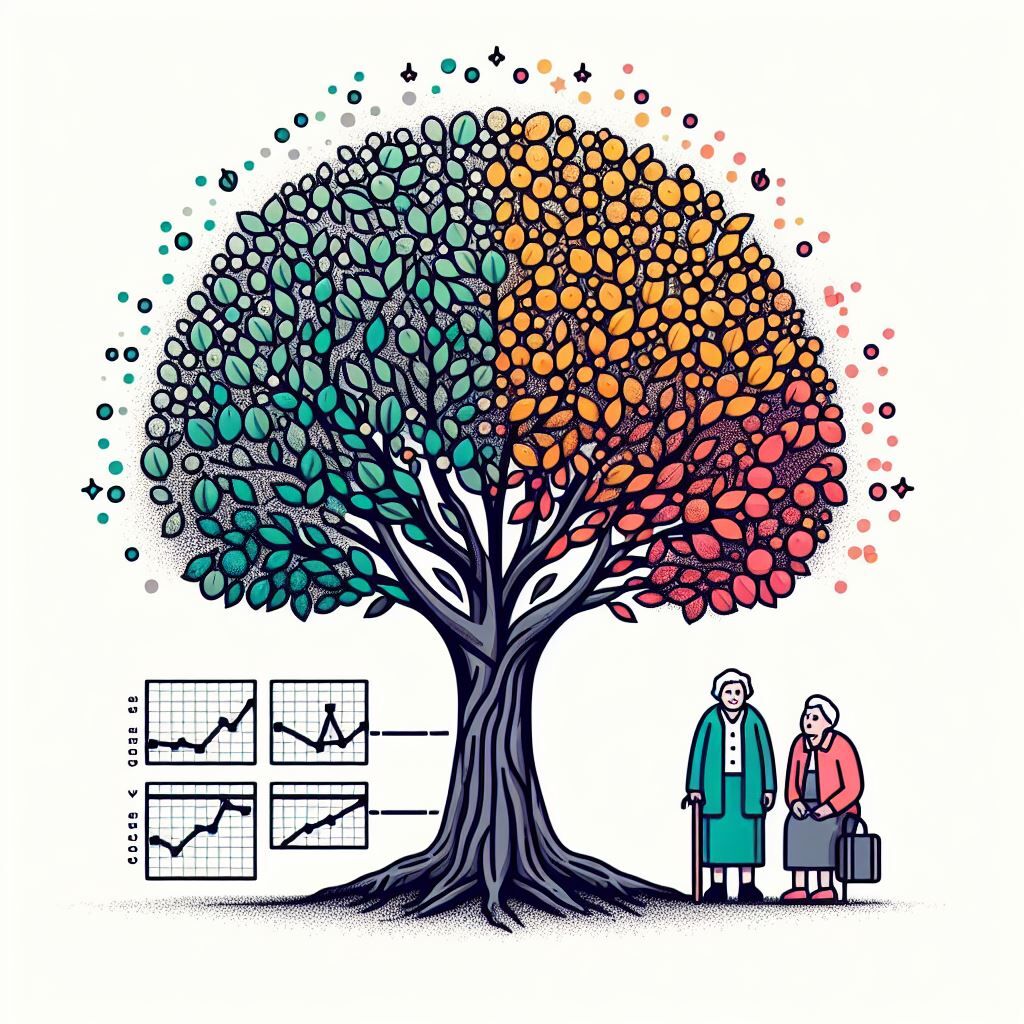
Downloads
Published
How to Cite
Issue
Section
License
Copyright (c) 2023 Revista Mexicana de Ingenieria Biomedica

This work is licensed under a Creative Commons Attribution-NonCommercial 4.0 International License.
Upon acceptance of an article in the RMIB, corresponding authors will be asked to fulfill and sign the copyright and the journal publishing agreement, which will allow the RMIB authorization to publish this document in any media without limitations and without any cost. Authors may reuse parts of the paper in other documents and reproduce part or all of it for their personal use as long as a bibliographic reference is made to the RMIB. However written permission of the Publisher is required for resale or distribution outside the corresponding author institution and for all other derivative works, including compilations and translations.