Pix2Pix Generative Adversarial Network for Cellular Nuclei and Cytoplasm Segmentation on Pap Smear Images
DOI:
https://doi.org/10.17488/RMIB.46.2.1462Keywords:
cancer, cGAN, segmentation, PAP, Pix2PixAbstract
In medical imaging for Pap smear tests, accurately identifying regions of interest, such as the nucleus and cytoplasm, remains a critical challenge due to the complex morphology and overlapping structures in cervical cell images. This complexity increases the risk of misidentification, potentially leading to false positives in computer-assisted diagnosis. To address this issue, this study introduces a novel approach by developing and evaluating a framework for the precise segmentation of nuclei and cytoplasm in cervical cell images using a cGAN-based model, Pix2Pix, applied to a dataset validated by specialists. The generated images are compared with target images, converted to binary, and an AND operation is performed to evaluate pixel overlap in the areas of interest. The evaluation metrics highlight a segmentation accuracy of 88.8 % and sensitivity of 89.62 % for nuclei, while for cytoplasm, precision reached 89.62 % and sensitivity 99.34 %. The Jaccard indices were 80.89 % for nuclei and 96.71 % for cytoplasm. These results demonstrate the effectiveness of the model in segmenting nuclei and cytoplasm in cervical cells.
Downloads
References
American Cancer Society. “What is cervical cancer?” Accessed: Nov. 05, 2023. [Online]. Available: https://www.cancer.org/cancer/types/cervical-cancer/about/what-is-cervical-cancer.html
World Health Organization. “Cervical cáncer.” Accessed: Jul. 10, 2024. [Online]. Available: https://www.who.int/news-room/fact-sheets/detail/cervical-cancer
The American Cancer Society. “Understanding what cancer is: ancient times to present.” Accessed: Jul. 11, 2024. [Online]. Available: https://www.cancer.org/cancer/understanding-cancer/history-of-cancer/what-is-cancer.html
National Cancer Institute. “Cervical cancer—health professional version.” Accessed: Jul. 10, 2024. [Online]. Available: https://www.cancer.gov/types/cervical/hp
The American Cancer Society, “Test for cervical Cancer” Accessed: Jul. 11, 2024. [Online]. Available: https://www.cancer.org/cancer/cervical-cancer/detection-diagnosis-staging/screening-tests.html
J. F. Barter, “The life and contributions of Doctor George Nicholas Papanicolaou,” Surg. Gynecol. Obstet., vol. 174, no. 6, pp. 530–532, 1992.
National Cancer Institute. “HPV and Pap test results: next steps after an abnormal cervical cancer screening test.” Accessed: Jul. 19, 2024. [Online]. Available: https://www.cancer.gov/types/cervical/screening/abnormal-hpv-pap-test-results
J.W. Sellors and R. Sankaranarayanan, Colposcopy and treatment of cervical intraepithelial neoplasia: a beginners manial, Cervical Cancer Screening - Chapter 2. (2003). Accessed: Aug. 20, 2024. [Online]. Available: https://screening.iarc.fr/colpochap.php?lang=3&chap=2
R. Nayar and D. C. Wilbur, The bethesda system for reporting cervical cytology: definitions, criteria, and explanatory notes, 3rd. Ed., Springer, 2015, doi: https://doi.org/10.1007/978-3-319-11074-5
R. A. Castellino, “Computer aided detection (CAD): an overview,” Cancer Imaging, vol. 5, no. 1, pp. 17–19, 2005, doi: https://doi.org/10.1102/1470-7330.2005.0018
P. Wang et al., “Automatic cell nuclei segmentation and classification of cervical Pap smear images,” Biomed. Signal Process Control, vol. 48, pp. 93–103, 2019, doi: https://doi.org/10.1016/j.bspc.2018.09.008
M. Zhao et al., “SEENS: Nuclei segmentation in Pap smear images with selective edge enhancement,” Future Gener. Comput. Syst., vol. 114, pp. 185–194, 2021, doi: https://doi.org/10.1016/j.future.2020.07.045
R. Saha, M. Bajger, and G. Lee, “Spatial shape constrained Fuzzy C-Means (FCM) clustering for nucleus segmentation in pap smear images,” in 2016 International Conference on Digital Image Computing: Techniques and Applications (DICTA), Gold Coast, QLD, Australia, 2016, pp. 1–8. doi: https://doi.org/10.1109/DICTA.2016.7797086
H. D. Cheng, X. H. Jiang, Y. Sun, and J. Wang, “Color image segmentation: advances and prospects,” Pattern Recognit., vol. 34, no. 12, pp. 2259–2281, 2001, doi: https://doi.org/10.1016/S0031-3203(00)00149-7
M. Lucic et al., “Are GANs created equal? a large-scale study,” 2017, arXiv: 1711.10337, doi: https://doi.org/10.48550/arXiv.1711.10337
S. Kato and K. Hotta, “Cell segmentation by image-to-image translation using multiple different discriminators,” in Proc. 13th Int. Joint Conf. Biomed. Eng. Sys. Technol., SCITEPRESS -Science and Technology Publications, Valletta, Malta, 2020, vol. 4, pp. 330–335, doi: https://doi.org/10.5220/0009170103300335
O. Ronneberger, P. Fischer, and T. Brox, “U-Net: Convolutional networks for biomedical image segmentation,” in 18th International Conference Medical Image Computing and Computer-Assisted Intervention – MICCAI 2015, in Lecture Notes in Computer Science (LNIP), vol. 9351, 2015, pp. 234–241, doi: https://doi.org/10.1007/978-3-319-24574-4_28
J. Zak et al., “Cell image augmentation for classification task using GANs on Pap smear dataset,” Biocybern. Biomed. Eng., vol. 42, no. 3, pp. 995–1011, 2022, doi: https://doi.org/10.1016/j.bbe.2022.07.003
S. Tahri et al., “A high-performance method of deep learning for prostate MR-only radiotherapy planning using an optimized Pix2Pix architecture,” Phys. Med., vol. 103, pp. 108–118, 2022, doi: https://doi.org/10.1016/j.ejmp.2022.10.003
Y. Xue et al., “Selective synthetic augmentation with HistoGAN for improved histopathology image classification,” Med. Image Anal., vol. 67, 2021, art. no. 101816, doi: https://doi.org/10.1016/j.media.2020.101816
W. A. Mustafa, A. Halim, M. A. Jamlos, and S. Z. S. Idrus, “A Review: Pap Smear Analysis Based on Image Processing Approach,” in 2nd Joint International Conference on Emerging Computing Technology and Sports (JICETS), Bandung, Indonesia, 2019, vol. 1529, art. no. 22080, doi: https://doi.org/10.1088/1742-6596/1529/2/022080
M. E. Plissiti et al., “Sipakmed: A New Dataset for Feature and Image Based Classification of Normal and Pathological Cervical Cells in Pap Smear Images,” in 2018 25th IEEE International Conference on Image Processing (ICIP), Athens, Greece, 2018, pp. 3144–3148, doi: https://doi.org/10.1109/ICIP.2018.8451588
P. Isola, J.-Y. Zhu, T. Zhou, and A. A. Efros, “Image-to-Image Translation with Conditional Adversarial Networks,” 2016, arXiv: 1611.07004, doi: https://doi.org/10.48550/arXiv.1611.07004
I. J. Goodfellow et al., “Generative Adversarial Networks,” 2014, arXiv: 1406.2661, doi: https://doi.org/10.48550/arXiv.1406.2661
M. Mirza and S. Osindero, “Conditional Generative Adversarial Nets,” 2014, arXiv: 1411.1784, doi: https://doi.org/10.48550/arXiv.1411.1784
D. M. W. Powers, “Evaluation: from precision, recall and F-measure to ROC, informedness, markedness and correlation,” 2020, arXiv: 2010.16061, doi: https://doi.org/10.48550/arXiv.2010.16061
M. Sokolova and G. Lapalme, “A systematic analysis of performance measures for classification tasks,” Inf. Process. Manag., vol. 45, no. 4, pp. 427–437, 2009, doi: https://doi.org/10.1016/j.ipm.2009.03.002
M. Neghina et al., “Automatic detection of cervical cells in Pap-smear images using polar transform and k-means segmentation,” in 2016 Sixth International Conference on Image Processing Theory, Tools and Applications (IPTA), Oulu, Finland, 2016, pp. 1–6, doi: https://doi.org/10.1109/IPTA.2016.7821038
Y. Song et al., “Accurate Cervical Cell Segmentation from Overlapping Clumps in Pap Smear Images,” IEEE Trans. Med. Imaging, vol. 36, no. 1, pp. 288–300, 2017, doi: https://doi.org/10.1109/TMI.2016.2606380
A. Desiani et al., “Bi-path architecture of CNN segmentation and classification method for cervical cancer disorders based on Pap-smear images.,” IAENG Int. J. Comput. Sci., vol. 48, no. 3, 2021. [Online]. Available: https://www.iaeng.org/IJCS/issues_v48/issue_3/IJCS_48_3_37.pdf
Y. Song et al., “A deep learning based framework for accurate segmentation of cervical cytoplasm and nuclei,” in 2014 36th Annual International Conference of the IEEE Engineering in Medicine and Biology Society, Chicago, IL, USA, 2014, pp. 2903–2906, doi: https://doi.org/10.1109/EMBC.2014.6944230
N. Nazir, A. Sarwar, B. S. Saini, and R. Shams, “A Robust deep learning approach for accurate segmentation of cytoplasm and nucleus in noisy pap smear images,” Computation, vol. 11, no. 10, 2023, art. no. 195, doi: https://doi.org/10.3390/computation11100195
C. Huang, X. Li, and Y. Wen, “AN OTSU image segmentation based on fruitfly optimization algorithm,” Alex. Eng. J., vol. 60, no. 1, pp. 183–188, 2021, doi: https://doi.org/10.1016/j.aej.2020.06.054
Y. Zhao et al., “Automatic segmentation of cervical cells based on star-convex polygons in Pap smear images,” Bioengineering, vol. 10, no. 1, 2022, art. no. 47, doi: https://doi.org/10.3390/bioengineering10010047
L. Zhang et al., “DeepPap: deep convolutional networks for cervical cell classification,” IEEE J. Biomed. Health Inform., vol. 21, no. 6, pp. 1633–1643, 2017, doi: https://doi.org/10.1109/JBHI.2017.2705583
B. Harangi et al., “Cell detection on digitized Pap smear images using ensemble of conventional image processing and deep learning techniques,” in 2019 11th International Symposium on Image and Signal Processing and Analysis (ISPA), Dubrovnik, Croatia, 2019, pp. 38–42, doi: https://doi.org/10.1109/ISPA.2019.8868683
M. J. Del Moral-Argumedo, C. A. Ochoa-Zezzati, R. Posada-Gómez, and A. A. Aguilar-Lasserre, “A deep learning approach for automated cytoplasm and nuclei cervical segmentation,” Biomed. Signal. Process. Control., vol. 81, 2023, art. no. 104483, doi: https://doi.org/10.1016/j.bspc.2022.104483
B. Z. Wubineh, A. Rusiecki, and K. Halawa, “Segmentation and classification techniques for Pap smear images in detecting cervical cancer: a systematic review,” IEEE Access, vol. 12, pp. 118195–118213, 2024, doi: https://doi.org/10.1109/ACCESS.2024.3447887
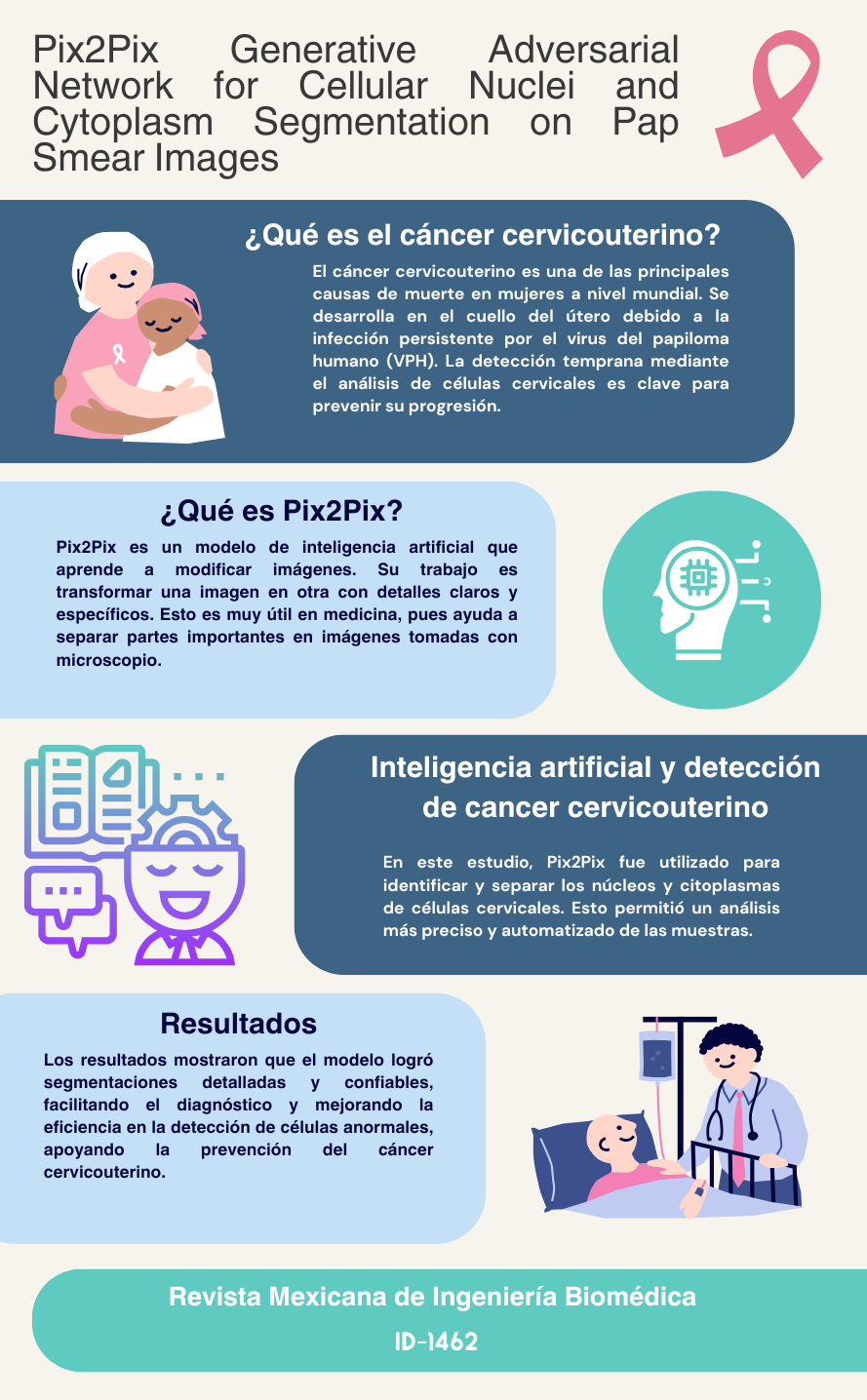
Downloads
Published
How to Cite
Issue
Section
License
Copyright (c) 2025 Revista Mexicana de Ingenieria Biomedica

This work is licensed under a Creative Commons Attribution-NonCommercial 4.0 International License.
Upon acceptance of an article in the RMIB, corresponding authors will be asked to fulfill and sign the copyright and the journal publishing agreement, which will allow the RMIB authorization to publish this document in any media without limitations and without any cost. Authors may reuse parts of the paper in other documents and reproduce part or all of it for their personal use as long as a bibliographic reference is made to the RMIB. However written permission of the Publisher is required for resale or distribution outside the corresponding author institution and for all other derivative works, including compilations and translations.