Red Generativa Antagónica Pix2Pix para la Segmentación de Núcleos Celulares y Citoplasma en Imágenes de Frotis de Papanicolaou
DOI:
https://doi.org/10.17488/RMIB.46.2.1462Palabras clave:
cáncer, cGAN, segmentación, PAP, Pix2PixResumen
En el campo de las imágenes médicas para la prueba de Papanicolaou, identificar con precisión las regiones de interés, como el núcleo y el citoplasma, sigue siendo un desafío crítico debido a la compleja morfología y las estructuras superpuestas en las imágenes de células cervicales. Esta complejidad aumenta el riesgo de identificaciones erróneas, lo que podría llevar a falsos positivos en el diagnóstico asistido por computadora. Para abordar este problema, este estudio presenta un enfoque novedoso mediante el desarrollo y evaluación de un marco para la segmentación precisa de núcleos y citoplasmas en imágenes de células cervicales, utilizando un modelo basado en cGAN, Pix2Pix, aplicado a un conjunto de datos validado por especialistas. Las imágenes generadas se compararon con las imágenes objetivo, se convirtieron a formato binario y se realizó una operación AND para evaluar la superposición de píxeles en las áreas de interés. Las métricas de evaluación destacaron una precisión de segmentación del 88.8 % y una sensibilidad del 89.62 % para los núcleos, mientras que, para el citoplasma, la precisión alcanzó el 89.62 % y la sensibilidad el 99.34 %. Los índices de Jaccard fueron del 80.89 % para los núcleos y del 96.71 % para el citoplasma. Estos resultados demuestran la efectividad del modelo en la segmentación de núcleos y citoplasmas en células cervicales.
Descargas
Citas
American Cancer Society. “What is cervical cancer?” Accessed: Nov. 05, 2023. [Online]. Available: https://www.cancer.org/cancer/types/cervical-cancer/about/what-is-cervical-cancer.html
World Health Organization. “Cervical cáncer.” Accessed: Jul. 10, 2024. [Online]. Available: https://www.who.int/news-room/fact-sheets/detail/cervical-cancer
The American Cancer Society. “Understanding what cancer is: ancient times to present.” Accessed: Jul. 11, 2024. [Online]. Available: https://www.cancer.org/cancer/understanding-cancer/history-of-cancer/what-is-cancer.html
National Cancer Institute. “Cervical cancer—health professional version.” Accessed: Jul. 10, 2024. [Online]. Available: https://www.cancer.gov/types/cervical/hp
The American Cancer Society, “Test for cervical Cancer” Accessed: Jul. 11, 2024. [Online]. Available: https://www.cancer.org/cancer/cervical-cancer/detection-diagnosis-staging/screening-tests.html
J. F. Barter, “The life and contributions of Doctor George Nicholas Papanicolaou,” Surg. Gynecol. Obstet., vol. 174, no. 6, pp. 530–532, 1992.
National Cancer Institute. “HPV and Pap test results: next steps after an abnormal cervical cancer screening test.” Accessed: Jul. 19, 2024. [Online]. Available: https://www.cancer.gov/types/cervical/screening/abnormal-hpv-pap-test-results
J.W. Sellors and R. Sankaranarayanan, Colposcopy and treatment of cervical intraepithelial neoplasia: a beginners manial, Cervical Cancer Screening - Chapter 2. (2003). Accessed: Aug. 20, 2024. [Online]. Available: https://screening.iarc.fr/colpochap.php?lang=3&chap=2
R. Nayar and D. C. Wilbur, The bethesda system for reporting cervical cytology: definitions, criteria, and explanatory notes, 3rd. Ed., Springer, 2015, doi: https://doi.org/10.1007/978-3-319-11074-5
R. A. Castellino, “Computer aided detection (CAD): an overview,” Cancer Imaging, vol. 5, no. 1, pp. 17–19, 2005, doi: https://doi.org/10.1102/1470-7330.2005.0018
P. Wang et al., “Automatic cell nuclei segmentation and classification of cervical Pap smear images,” Biomed. Signal Process Control, vol. 48, pp. 93–103, 2019, doi: https://doi.org/10.1016/j.bspc.2018.09.008
M. Zhao et al., “SEENS: Nuclei segmentation in Pap smear images with selective edge enhancement,” Future Gener. Comput. Syst., vol. 114, pp. 185–194, 2021, doi: https://doi.org/10.1016/j.future.2020.07.045
R. Saha, M. Bajger, and G. Lee, “Spatial shape constrained Fuzzy C-Means (FCM) clustering for nucleus segmentation in pap smear images,” in 2016 International Conference on Digital Image Computing: Techniques and Applications (DICTA), Gold Coast, QLD, Australia, 2016, pp. 1–8. doi: https://doi.org/10.1109/DICTA.2016.7797086
H. D. Cheng, X. H. Jiang, Y. Sun, and J. Wang, “Color image segmentation: advances and prospects,” Pattern Recognit., vol. 34, no. 12, pp. 2259–2281, 2001, doi: https://doi.org/10.1016/S0031-3203(00)00149-7
M. Lucic et al., “Are GANs created equal? a large-scale study,” 2017, arXiv: 1711.10337, doi: https://doi.org/10.48550/arXiv.1711.10337
S. Kato and K. Hotta, “Cell segmentation by image-to-image translation using multiple different discriminators,” in Proc. 13th Int. Joint Conf. Biomed. Eng. Sys. Technol., SCITEPRESS -Science and Technology Publications, Valletta, Malta, 2020, vol. 4, pp. 330–335, doi: https://doi.org/10.5220/0009170103300335
O. Ronneberger, P. Fischer, and T. Brox, “U-Net: Convolutional networks for biomedical image segmentation,” in 18th International Conference Medical Image Computing and Computer-Assisted Intervention – MICCAI 2015, in Lecture Notes in Computer Science (LNIP), vol. 9351, 2015, pp. 234–241, doi: https://doi.org/10.1007/978-3-319-24574-4_28
J. Zak et al., “Cell image augmentation for classification task using GANs on Pap smear dataset,” Biocybern. Biomed. Eng., vol. 42, no. 3, pp. 995–1011, 2022, doi: https://doi.org/10.1016/j.bbe.2022.07.003
S. Tahri et al., “A high-performance method of deep learning for prostate MR-only radiotherapy planning using an optimized Pix2Pix architecture,” Phys. Med., vol. 103, pp. 108–118, 2022, doi: https://doi.org/10.1016/j.ejmp.2022.10.003
Y. Xue et al., “Selective synthetic augmentation with HistoGAN for improved histopathology image classification,” Med. Image Anal., vol. 67, 2021, art. no. 101816, doi: https://doi.org/10.1016/j.media.2020.101816
W. A. Mustafa, A. Halim, M. A. Jamlos, and S. Z. S. Idrus, “A Review: Pap Smear Analysis Based on Image Processing Approach,” in 2nd Joint International Conference on Emerging Computing Technology and Sports (JICETS), Bandung, Indonesia, 2019, vol. 1529, art. no. 22080, doi: https://doi.org/10.1088/1742-6596/1529/2/022080
M. E. Plissiti et al., “Sipakmed: A New Dataset for Feature and Image Based Classification of Normal and Pathological Cervical Cells in Pap Smear Images,” in 2018 25th IEEE International Conference on Image Processing (ICIP), Athens, Greece, 2018, pp. 3144–3148, doi: https://doi.org/10.1109/ICIP.2018.8451588
P. Isola, J.-Y. Zhu, T. Zhou, and A. A. Efros, “Image-to-Image Translation with Conditional Adversarial Networks,” 2016, arXiv: 1611.07004, doi: https://doi.org/10.48550/arXiv.1611.07004
I. J. Goodfellow et al., “Generative Adversarial Networks,” 2014, arXiv: 1406.2661, doi: https://doi.org/10.48550/arXiv.1406.2661
M. Mirza and S. Osindero, “Conditional Generative Adversarial Nets,” 2014, arXiv: 1411.1784, doi: https://doi.org/10.48550/arXiv.1411.1784
D. M. W. Powers, “Evaluation: from precision, recall and F-measure to ROC, informedness, markedness and correlation,” 2020, arXiv: 2010.16061, doi: https://doi.org/10.48550/arXiv.2010.16061
M. Sokolova and G. Lapalme, “A systematic analysis of performance measures for classification tasks,” Inf. Process. Manag., vol. 45, no. 4, pp. 427–437, 2009, doi: https://doi.org/10.1016/j.ipm.2009.03.002
M. Neghina et al., “Automatic detection of cervical cells in Pap-smear images using polar transform and k-means segmentation,” in 2016 Sixth International Conference on Image Processing Theory, Tools and Applications (IPTA), Oulu, Finland, 2016, pp. 1–6, doi: https://doi.org/10.1109/IPTA.2016.7821038
Y. Song et al., “Accurate Cervical Cell Segmentation from Overlapping Clumps in Pap Smear Images,” IEEE Trans. Med. Imaging, vol. 36, no. 1, pp. 288–300, 2017, doi: https://doi.org/10.1109/TMI.2016.2606380
A. Desiani et al., “Bi-path architecture of CNN segmentation and classification method for cervical cancer disorders based on Pap-smear images.,” IAENG Int. J. Comput. Sci., vol. 48, no. 3, 2021. [Online]. Available: https://www.iaeng.org/IJCS/issues_v48/issue_3/IJCS_48_3_37.pdf
Y. Song et al., “A deep learning based framework for accurate segmentation of cervical cytoplasm and nuclei,” in 2014 36th Annual International Conference of the IEEE Engineering in Medicine and Biology Society, Chicago, IL, USA, 2014, pp. 2903–2906, doi: https://doi.org/10.1109/EMBC.2014.6944230
N. Nazir, A. Sarwar, B. S. Saini, and R. Shams, “A Robust deep learning approach for accurate segmentation of cytoplasm and nucleus in noisy pap smear images,” Computation, vol. 11, no. 10, 2023, art. no. 195, doi: https://doi.org/10.3390/computation11100195
C. Huang, X. Li, and Y. Wen, “AN OTSU image segmentation based on fruitfly optimization algorithm,” Alex. Eng. J., vol. 60, no. 1, pp. 183–188, 2021, doi: https://doi.org/10.1016/j.aej.2020.06.054
Y. Zhao et al., “Automatic segmentation of cervical cells based on star-convex polygons in Pap smear images,” Bioengineering, vol. 10, no. 1, 2022, art. no. 47, doi: https://doi.org/10.3390/bioengineering10010047
L. Zhang et al., “DeepPap: deep convolutional networks for cervical cell classification,” IEEE J. Biomed. Health Inform., vol. 21, no. 6, pp. 1633–1643, 2017, doi: https://doi.org/10.1109/JBHI.2017.2705583
B. Harangi et al., “Cell detection on digitized Pap smear images using ensemble of conventional image processing and deep learning techniques,” in 2019 11th International Symposium on Image and Signal Processing and Analysis (ISPA), Dubrovnik, Croatia, 2019, pp. 38–42, doi: https://doi.org/10.1109/ISPA.2019.8868683
M. J. Del Moral-Argumedo, C. A. Ochoa-Zezzati, R. Posada-Gómez, and A. A. Aguilar-Lasserre, “A deep learning approach for automated cytoplasm and nuclei cervical segmentation,” Biomed. Signal. Process. Control., vol. 81, 2023, art. no. 104483, doi: https://doi.org/10.1016/j.bspc.2022.104483
B. Z. Wubineh, A. Rusiecki, and K. Halawa, “Segmentation and classification techniques for Pap smear images in detecting cervical cancer: a systematic review,” IEEE Access, vol. 12, pp. 118195–118213, 2024, doi: https://doi.org/10.1109/ACCESS.2024.3447887
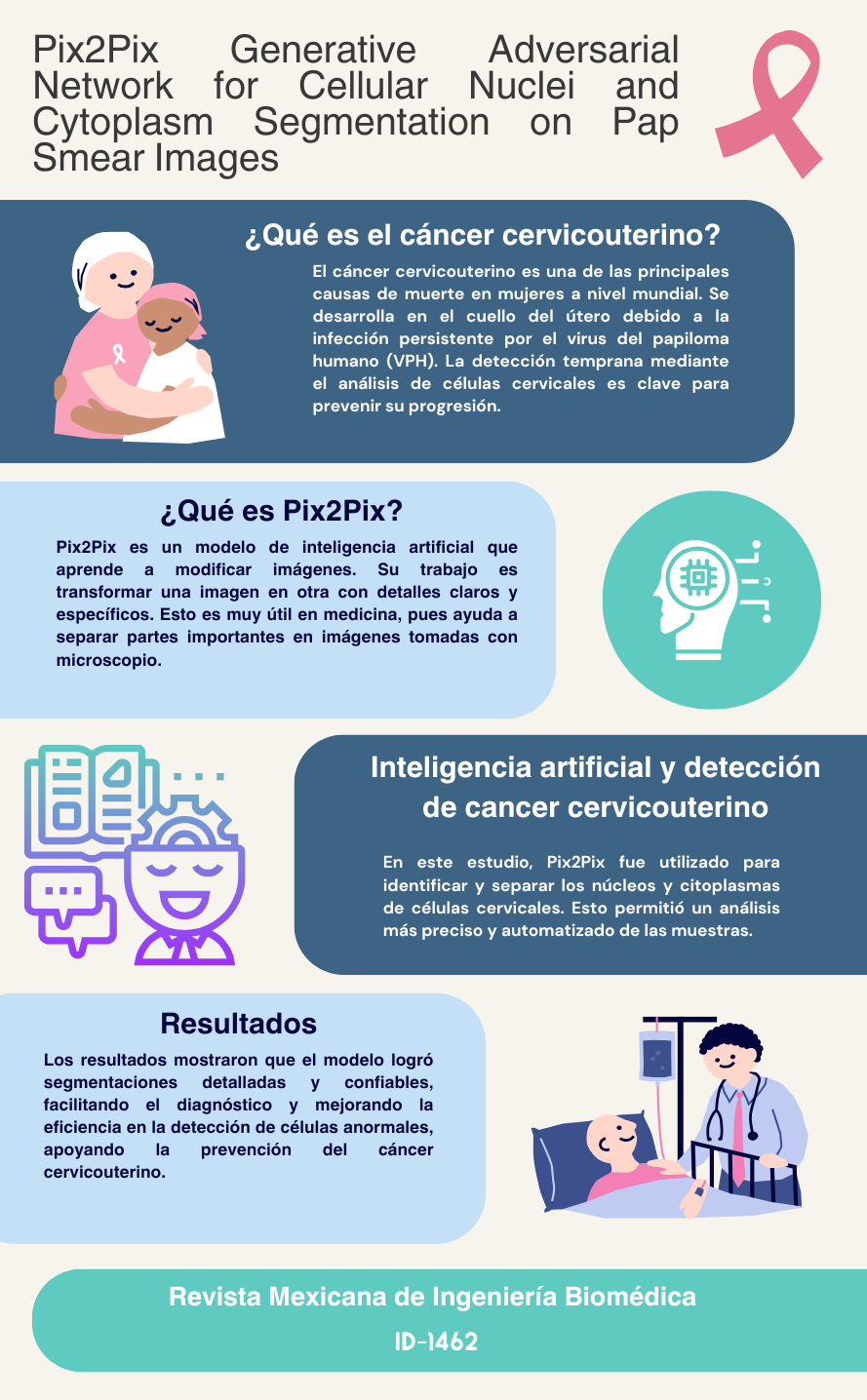
Descargas
Publicado
Cómo citar
Número
Sección
Licencia
Derechos de autor 2025 Revista Mexicana de Ingenieria Biomedica

Esta obra está bajo una licencia internacional Creative Commons Atribución-NoComercial 4.0.
Una vez que el artículo es aceptado para su publicación en la RMIB, se les solicitará al autor principal o de correspondencia que revisen y firman las cartas de cesión de derechos correspondientes para llevar a cabo la autorización para la publicación del artículo. En dicho documento se autoriza a la RMIB a publicar, en cualquier medio sin limitaciones y sin ningún costo. Los autores pueden reutilizar partes del artículo en otros documentos y reproducir parte o la totalidad para su uso personal siempre que se haga referencia bibliográfica al RMIB. No obstante, todo tipo de publicación fuera de las publicaciones académicas del autor correspondiente o para otro tipo de trabajos derivados y publicados necesitaran de un permiso escrito de la RMIB.