Gammatone-Frequency Cepstral Coefficients Based Fear Emotion Level Recognition System
DOI:
https://doi.org/10.17488/RMIB.45.2.1Keywords:
fear emotion, gammatone-frequency cepstral coefficients, Mel-frequency cepstral coefficients, signal-to-noise reduction, speech soundAbstract
Emotions represent affective states that induce alterations in behavior and interactions within one's environment. An avenue for discerning human emotions lies in the realm of speech analysis. Empirical evidence indicates that 1.6 million Indonesian teenagers grapple with mental anxiety disorders, characterized by sensations of fear or ambiguous vigilance. This work endeavors to devise a tool for discerning an individual's emotional state through voice processing, focusing particularly on fear emotions stratified into three levels of intensity: low, medium, and high. The proposed system employs Gammatone-Frequency Cepstral Coefficients (GFCC) for feature extraction, leveraging the efficacy of its gamma filter in reducing noise. Furthermore, a Random Forest (RF) Classifier is integrated to facilitate the recognition of fear's emotional intensity in speech signals. The system is deployed on a Raspberry Pi 4B and establishes a Bluetooth connection using the RFCOMM communication protocol to an Android application, presenting the classification results. The outcomes reveal that the Signal-to-Noise Reduction achieved through GFCC extraction surpasses that of Mel-Frequency Cepstral Coefficients (MFCC). In terms of accuracy, the implemented recognition system for fear emotion levels, employing GFCC extraction and Random Forest Classifier, attains a commendable accuracy of 73.33 %.
Downloads
References
M. Gupta, S. S. Bharti, and S. Agarwal, “Gender-based speaker recognition from speech signals using GMM model,” Mod. Phys. Lett. B, vol. 33, no. 35, 2019, doi: https://doi.org/10.1142/S0217984919504384
J. Otašević and B. Otašević, “Voice-based identification and contribution to the efficiency of criminal proceedings,” J. Crim. Crim. Law, vol. 59, no. 2, pp. 61–72, Nov. 2021, doi: https://doi.org/10.47152/rkkp.59.2.4
S. A. Kotz, R. Dengler, and M. Wittfoth, “Valence-specific conflict moderation in the dorso-medial PFC and the caudate head in emotional speech,” Soc. Cogn. Affect Neurosci., vol. 10, no. 2, 2015, doi: https://doi.org/10.1093/scan/nsu021
S. J. Gomez, “Self-Management Skills of Management Graduates,” Int. J. Res. Manag. Bus. Stud., vol. 4, no. 3, pp. 40-44, 2017.
S. A. Saddiqui, M. Jawad, M. Naz, and G. S. Khan Niazi, “Emotional intelligence and managerial effectiveness,” RIC, vol. 4, no. 1, pp. 99-130, 2018, doi: https://doi.org/10.32728/RIC.2018.41%2F5
H. E. Erskine, S. J. Blondell, M. E. Enright, J. Shadid, et al., “Measuring the Prevalence of Mental Disorders in Adolescents in Kenya, Indonesia, and Vietnam: Study Protocol for the National Adolescent Mental Health Surveys,” J. Adolesc. Health, vol. 72, no. 1, pp. S71-S78, 2023, doi: https://doi.org/10.1016/j.jadohealth.2021.05.012
K. Cherry, “What Are Emotions and the Types of Emotional Responses?” Verywell Health. https://www.verywellhealth.com/what-are-emotions-279517807 (accessed 2023).
S. Sharma, A. Mamata, Deepak, “Psychological Impacts, Hand Hygeine Practices & and Its Correlates in View of Covid-19 among Health Care Professionals in Northern States of India,” Indian J. Forensic Med. Toxicol., vol. 15, no. 2, pp. 3691-3698, 2021, doi: https://doi.org/10.37506/ijfmt.v15i2.14947
S. A. Mahar, M. H. Mahar, J. A. Mahar, M. Masud, M. Ahmad, N. Z. Jhanhi, and M. A. Razzaq, “Superposition of functional contours based prosodic feature extraction for speech processing,” Intell. Autom. Soft Comput., vol. 29, no. 1, pp. 183-197, 2021, doi: https://doi.org/10.32604/iasc.2021.015755
S. Sondhi, M. Khan, R. Vijay, A. K. Salhan, and S. Chouhan, “Acoustic analysis of speech under stress,” Int. J. Bioinform. Res. Appl., vol. 11, no. 5, pp. 417-432, 2015, doi: https://doi.org/10.1504/ijbra.2015.071942
M. S. Likitha, S. R. R. Gupta, K. Hasitha, and A. U. Raju, “Speech based human emotion recognition using MFCC,” in 2017 International Conference on Wireless Communications, Signal Processing and Networking (WiSPNET), Chennai, India, 2017, pp. 2257-2260, doi: https://doi.org/10.1109/WiSPNET.2017.8300161
M. Jeevan, A. Dhingra, M. Hanmandlu, and B. K. Panigrahi, “Robust speaker verification using GFCC based i-vectors,” in Proceedings of the International Conference on Signal, Networks, Computing, and Systems. Lecture Notes in Electrical Engineering, vol 395. New Delhi, India, pp. 85-91, 2017, doi: https://doi.org/10.1007/978-81-322-3592-7_9
H. Wang and C. Zhang, “The application of Gammatone frequency cepstral coefficients for forensic voice comparison under noisy conditions,” Aust. J. Forensic Sci., vol. 52, no. 5, pp. 553-568, 2020, doi: https://doi.org/10.1080/00450618.2019.1584830
D. Bharti and P. Kukana, “A Hybrid Machine Learning Model for Emotion Recognition from Speech Signals,” in 2020 International Conference on Smart Electronics and Communication (ICOSEC), Trichy, India, 2020, pp. 491-496, doi: https://doi.org/10.1109/ICOSEC49089.2020.9215376
H. Patni, A. Jagtap, V. Bhoyar, and A. Gupta, “Speech Emotion Recognition using MFCC, GFCC, Chromagram and RMSE features,” in 2021 8th International Conference on Signal Processing and Integrated Networks (SPIN), Noida, India, 2021, pp. 892-897, doi: https://doi.org/10.1109/SPIN52536.2021.9566046
H. Choudhary, D. Sadhya, and V. Patel, “Automatic Speaker Verification using Gammatone Frequency Cepstral Coefficients,” in 2021 8th International Conference on Signal Processing and Integrated Networks (SPIN), Noida, India, 2021, pp. 424-428, doi: https://doi.org/10.1109/SPIN52536.2021.9566150
L. Zheng, Q. Li, H. Ban, and S. Liu, “Speech emotion recognition based on convolution neural network combined with random forest,” in 2018 Chinese Control And Decision Conference (CCDC), Shenyang, China, 2018, pp. 4143-4147, doi: https://doi.org/10.1109/CCDC.2018.8407844
S. Hamsa, I. Shahin, Y. Iraqi, and N. Werghi, “Emotion Recognition from Speech Using Wavelet Packet Transform Cochlear Filter Bank and Random Forest Classifier,” IEEE Access, vol. 8, pp. 96994-97006, 2020, doi: https://doi.org/10.1109/ACCESS.2020.2991811
A. Cuncic, “Amygdala Hijack and the Fight or Flight Response,” Very Well Mind. https://www.verywellmind.com/what-happens-during-an-amygdala-hijack-4165944 (accessed 2023).
E. B. Foa and M. J. Kozak, “Emotional Processing of Fear. Exposure to Corrective Information,” Psychol. Bull., vol. 99, no. 1, pp. 20-35, 1986, doi: https://psycnet.apa.org/doi/10.1037/0033-2909.99.1.20
S. M. Qaisar, “Isolated speech recognition and its transformation in visual signs,” J. Electr. Eng. Technol., vol. 14, no. 2, pp. 955-964, 2019, doi: https://doi.org/10.1007/s42835-018-00071-z
S. Lokesh and M. R. Devi, “Speech recognition system using enhanced mel frequency cepstral coefficient with windowing and framing method,” Cluster Comput., vol. 22, pp. 11669-11679, 2019, doi: https://doi.org/10.1007/s10586-017-1447-6
J. D. Schmidt, “Simple Computations Using Fourier Transforms,” in Numerical Simulation of Optical Wave Propagation with Examples in MATLAB, Bellingham, WA, USA: SPIE Press, 2010, doi: https://doi.org/10.1117/3.866274.ch3
A. Krobba, M. Debyeche, and S. A. Selouani, “Mixture linear prediction Gammatone Cepstral features for robust speaker verification under transmission channel noise,” Multimed. Tools Appl., vol. 79, no. 25–26, pp. 18679-18693, 2020, doi: https://doi.org/10.1007/s11042-020-08748-2
A. Revathi, N. Sasikaladevi, R. Nagakrishnan, and C. Jeyalakshmi, “Robust emotion recognition from speech: Gamma tone features and models,” Int. J. Speech Technol., vol. 21, no. 3, pp. 723-739, 2018, doi: https://doi.org/10.1007/s10772-018-9546-1
U. Kumaran, S. Radha Rammohan, S. M. Nagarajan, and A. Prathik, “Fusion of mel and gammatone frequency cepstral coefficients for speech emotion recognition using deep C-RNN,” Int. J. Speech Technol., vol. 24, no. 2, pp. 303-314, 2021, doi: https://doi.org/10.1007/s10772-020-09792-x
S. Rhee, M. G. Kang, “Discrete cosine transform based regularized high-resolution image reconstruction algorithm,” Opt. Eng., vol. 38, no. 8, pp. 1348-1356, 1999, doi: https://doi.org/10.1117/1.602177
A. Subudhi, M. Dash, and S. Sabut, “Automated segmentation and classification of brain stroke using expectation-maximization and random forest classifier,” Biocybern. Biomed. Eng., vol. 40, no. 1, pp. 277-289, 2020, doi: https://doi.org/10.1016/j.bbe.2019.04.004
T. N. Phan, V. Kuch, and L. W. Lehnert, “Land cover classification using google earth engine and random forest classifier-the role of image composition,” Remote Sens., vol. 12, no. 15, art. no. 2411, 2020, doi: https://doi.org/10.3390/rs12152411
T. Adiono, S. F. Anindya, S. Fuada, K. Afifah, and I. G. Purwanda, “Efficient Android Software Development Using MIT App Inventor 2 for Bluetooth-Based Smart Home,” Wireless Pers. Commun., vol. 105, pp. 233-256, 2019, doi: https://doi.org/10.1007/s11277-018-6110-x
H. Cao, D. G. Cooper, M. K. Keutmann, R. C. Gur, A. Nenkova, and R. Verma, “CREMA-D: Crowd-sourced Emotional Multimodal Actors Dataset,” IEEE Trans. Affect. Comput., vol. 5, no. 4, pp. 377-390, 2014, doi: https://doi.org/10.1109/taffc.2014.2336244
A. Mishra, D. Patil, N. Karkhanis, V. Gaikar, and K. Wani, “Real time emotion detection from speech using Raspberry Pi 3,” in 2017 International Conference on Wireless Communications, Signal Processing and Networking (WiSPNET), Chennai, India, 2017, pp. 2300-2303, doi: https://doi.org/10.1109/WiSPNET.2017.8300170
H. Alshamsi, V. Kepuska, H. Alshamsi, and H. Meng, “Automated Speech Emotion Recognition on Smart Phones,” in 2018 9th IEEE Annual Ubiquitous Computing, Electronics and Mobile Communication Conference (UEMCON), New York, NY, USA, 2018, pp. 44-50, doi: https://doi.org/10.1109/UEMCON.2018.8796594
S. Chebbi and S. Ben Jebara, “On the Selection of Relevant Features for Fear Emotion Detection from Speech,” in 2018 9th International Symposium on Signal, Image, Video and Communications (ISIVC), Rabat, Morocco, 2018, pp. 82-86, doi: https://doi.org/10.1109/ISIVC.2018.8709233
C. Clavel, I. Vasilescu, L. Devillers, G. Richard, and T. Ehrette, “Fear-type emotion recognition for future audio-based surveillance systems,” Speech Commun., vol. 50, no. 6, pp. 487-503, 2008, doi: https://doi.org/10.1016/j.specom.2008.03.012
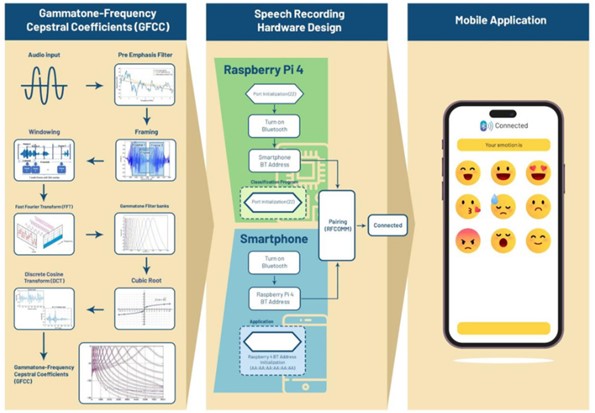
Published
How to Cite
Issue
Section
License
Copyright (c) 2024 Revista Mexicana de Ingenieria Biomedica

This work is licensed under a Creative Commons Attribution-NonCommercial 4.0 International License.
Upon acceptance of an article in the RMIB, corresponding authors will be asked to fulfill and sign the copyright and the journal publishing agreement, which will allow the RMIB authorization to publish this document in any media without limitations and without any cost. Authors may reuse parts of the paper in other documents and reproduce part or all of it for their personal use as long as a bibliographic reference is made to the RMIB. However written permission of the Publisher is required for resale or distribution outside the corresponding author institution and for all other derivative works, including compilations and translations.