Sistema de Reconocimiento de Nivel de Emoción Basado en Coeficientes Cepstrales de Frecuencia Gammatone
DOI:
https://doi.org/10.17488/RMIB.45.2.1Palabras clave:
emoción de miedo, coeficientes cepstrales de frecuencia gammatone, coeficientes cepstrales de frecuencia Mel, reducción de señal a ruido, sonido del hablaResumen
Las emociones representan estados afectivos que inducen alteraciones en el comportamiento e interacciones dentro del entorno de un individuo. Un enfoque para discernir las emociones humanas se encuentra en el análisis del habla. La evidencia empírica indica que 1.6 millones de adolescentes indonesios enfrentan trastornos de ansiedad mental, caracterizados por sensaciones de miedo o vigilancia ambigua. Esta investigación se propone diseñar una herramienta para discernir el estado emocional de una persona mediante el procesamiento de la voz, centrándose especialmente en las emociones de miedo estratificadas en tres niveles de intensidad: bajo, medio y alto. La metodología propuesta emplea los Coeficientes Cepstrales de Frecuencia Gammatone (GFCC) para la extracción de características, aprovechando la eficacia de su filtro gamma para combatir el ruido. Además, se incorpora un Clasificador Random Forest (RF) para facilitar el reconocimiento de la intensidad emocional del miedo en las señales de voz. El sistema se implementa en una Raspberry Pi 4B y establece una conexión Bluetooth utilizando el protocolo de comunicación RFCOMM con una aplicación Android, presentando los resultados de la clasificación. Los resultados revelan que la Reducción de Señal a Ruido lograda mediante la extracción de GFCC supera a la de los Coeficientes Cepstrales de Frecuencia Mel (MFCC). En términos de precisión, el sistema de reconocimiento implementado para los niveles de emoción de miedo, utilizando la extracción de GFCC y el Clasificador Random Forest, alcanza una precisión destacada del 73.33 %.
Descargas
Citas
M. Gupta, S. S. Bharti, and S. Agarwal, “Gender-based speaker recognition from speech signals using GMM model,” Mod. Phys. Lett. B, vol. 33, no. 35, 2019, doi: https://doi.org/10.1142/S0217984919504384
J. Otašević and B. Otašević, “Voice-based identification and contribution to the efficiency of criminal proceedings,” J. Crim. Crim. Law, vol. 59, no. 2, pp. 61–72, Nov. 2021, doi: https://doi.org/10.47152/rkkp.59.2.4
S. A. Kotz, R. Dengler, and M. Wittfoth, “Valence-specific conflict moderation in the dorso-medial PFC and the caudate head in emotional speech,” Soc. Cogn. Affect Neurosci., vol. 10, no. 2, 2015, doi: https://doi.org/10.1093/scan/nsu021
S. J. Gomez, “Self-Management Skills of Management Graduates,” Int. J. Res. Manag. Bus. Stud., vol. 4, no. 3, pp. 40-44, 2017.
S. A. Saddiqui, M. Jawad, M. Naz, and G. S. Khan Niazi, “Emotional intelligence and managerial effectiveness,” RIC, vol. 4, no. 1, pp. 99-130, 2018, doi: https://doi.org/10.32728/RIC.2018.41%2F5
H. E. Erskine, S. J. Blondell, M. E. Enright, J. Shadid, et al., “Measuring the Prevalence of Mental Disorders in Adolescents in Kenya, Indonesia, and Vietnam: Study Protocol for the National Adolescent Mental Health Surveys,” J. Adolesc. Health, vol. 72, no. 1, pp. S71-S78, 2023, doi: https://doi.org/10.1016/j.jadohealth.2021.05.012
K. Cherry, “What Are Emotions and the Types of Emotional Responses?” Verywell Health. https://www.verywellhealth.com/what-are-emotions-279517807 (accessed 2023).
S. Sharma, A. Mamata, Deepak, “Psychological Impacts, Hand Hygeine Practices & and Its Correlates in View of Covid-19 among Health Care Professionals in Northern States of India,” Indian J. Forensic Med. Toxicol., vol. 15, no. 2, pp. 3691-3698, 2021, doi: https://doi.org/10.37506/ijfmt.v15i2.14947
S. A. Mahar, M. H. Mahar, J. A. Mahar, M. Masud, M. Ahmad, N. Z. Jhanhi, and M. A. Razzaq, “Superposition of functional contours based prosodic feature extraction for speech processing,” Intell. Autom. Soft Comput., vol. 29, no. 1, pp. 183-197, 2021, doi: https://doi.org/10.32604/iasc.2021.015755
S. Sondhi, M. Khan, R. Vijay, A. K. Salhan, and S. Chouhan, “Acoustic analysis of speech under stress,” Int. J. Bioinform. Res. Appl., vol. 11, no. 5, pp. 417-432, 2015, doi: https://doi.org/10.1504/ijbra.2015.071942
M. S. Likitha, S. R. R. Gupta, K. Hasitha, and A. U. Raju, “Speech based human emotion recognition using MFCC,” in 2017 International Conference on Wireless Communications, Signal Processing and Networking (WiSPNET), Chennai, India, 2017, pp. 2257-2260, doi: https://doi.org/10.1109/WiSPNET.2017.8300161
M. Jeevan, A. Dhingra, M. Hanmandlu, and B. K. Panigrahi, “Robust speaker verification using GFCC based i-vectors,” in Proceedings of the International Conference on Signal, Networks, Computing, and Systems. Lecture Notes in Electrical Engineering, vol 395. New Delhi, India, pp. 85-91, 2017, doi: https://doi.org/10.1007/978-81-322-3592-7_9
H. Wang and C. Zhang, “The application of Gammatone frequency cepstral coefficients for forensic voice comparison under noisy conditions,” Aust. J. Forensic Sci., vol. 52, no. 5, pp. 553-568, 2020, doi: https://doi.org/10.1080/00450618.2019.1584830
D. Bharti and P. Kukana, “A Hybrid Machine Learning Model for Emotion Recognition from Speech Signals,” in 2020 International Conference on Smart Electronics and Communication (ICOSEC), Trichy, India, 2020, pp. 491-496, doi: https://doi.org/10.1109/ICOSEC49089.2020.9215376
H. Patni, A. Jagtap, V. Bhoyar, and A. Gupta, “Speech Emotion Recognition using MFCC, GFCC, Chromagram and RMSE features,” in 2021 8th International Conference on Signal Processing and Integrated Networks (SPIN), Noida, India, 2021, pp. 892-897, doi: https://doi.org/10.1109/SPIN52536.2021.9566046
H. Choudhary, D. Sadhya, and V. Patel, “Automatic Speaker Verification using Gammatone Frequency Cepstral Coefficients,” in 2021 8th International Conference on Signal Processing and Integrated Networks (SPIN), Noida, India, 2021, pp. 424-428, doi: https://doi.org/10.1109/SPIN52536.2021.9566150
L. Zheng, Q. Li, H. Ban, and S. Liu, “Speech emotion recognition based on convolution neural network combined with random forest,” in 2018 Chinese Control And Decision Conference (CCDC), Shenyang, China, 2018, pp. 4143-4147, doi: https://doi.org/10.1109/CCDC.2018.8407844
S. Hamsa, I. Shahin, Y. Iraqi, and N. Werghi, “Emotion Recognition from Speech Using Wavelet Packet Transform Cochlear Filter Bank and Random Forest Classifier,” IEEE Access, vol. 8, pp. 96994-97006, 2020, doi: https://doi.org/10.1109/ACCESS.2020.2991811
A. Cuncic, “Amygdala Hijack and the Fight or Flight Response,” Very Well Mind. https://www.verywellmind.com/what-happens-during-an-amygdala-hijack-4165944 (accessed 2023).
E. B. Foa and M. J. Kozak, “Emotional Processing of Fear. Exposure to Corrective Information,” Psychol. Bull., vol. 99, no. 1, pp. 20-35, 1986, doi: https://psycnet.apa.org/doi/10.1037/0033-2909.99.1.20
S. M. Qaisar, “Isolated speech recognition and its transformation in visual signs,” J. Electr. Eng. Technol., vol. 14, no. 2, pp. 955-964, 2019, doi: https://doi.org/10.1007/s42835-018-00071-z
S. Lokesh and M. R. Devi, “Speech recognition system using enhanced mel frequency cepstral coefficient with windowing and framing method,” Cluster Comput., vol. 22, pp. 11669-11679, 2019, doi: https://doi.org/10.1007/s10586-017-1447-6
J. D. Schmidt, “Simple Computations Using Fourier Transforms,” in Numerical Simulation of Optical Wave Propagation with Examples in MATLAB, Bellingham, WA, USA: SPIE Press, 2010, doi: https://doi.org/10.1117/3.866274.ch3
A. Krobba, M. Debyeche, and S. A. Selouani, “Mixture linear prediction Gammatone Cepstral features for robust speaker verification under transmission channel noise,” Multimed. Tools Appl., vol. 79, no. 25–26, pp. 18679-18693, 2020, doi: https://doi.org/10.1007/s11042-020-08748-2
A. Revathi, N. Sasikaladevi, R. Nagakrishnan, and C. Jeyalakshmi, “Robust emotion recognition from speech: Gamma tone features and models,” Int. J. Speech Technol., vol. 21, no. 3, pp. 723-739, 2018, doi: https://doi.org/10.1007/s10772-018-9546-1
U. Kumaran, S. Radha Rammohan, S. M. Nagarajan, and A. Prathik, “Fusion of mel and gammatone frequency cepstral coefficients for speech emotion recognition using deep C-RNN,” Int. J. Speech Technol., vol. 24, no. 2, pp. 303-314, 2021, doi: https://doi.org/10.1007/s10772-020-09792-x
S. Rhee, M. G. Kang, “Discrete cosine transform based regularized high-resolution image reconstruction algorithm,” Opt. Eng., vol. 38, no. 8, pp. 1348-1356, 1999, doi: https://doi.org/10.1117/1.602177
A. Subudhi, M. Dash, and S. Sabut, “Automated segmentation and classification of brain stroke using expectation-maximization and random forest classifier,” Biocybern. Biomed. Eng., vol. 40, no. 1, pp. 277-289, 2020, doi: https://doi.org/10.1016/j.bbe.2019.04.004
T. N. Phan, V. Kuch, and L. W. Lehnert, “Land cover classification using google earth engine and random forest classifier-the role of image composition,” Remote Sens., vol. 12, no. 15, art. no. 2411, 2020, doi: https://doi.org/10.3390/rs12152411
T. Adiono, S. F. Anindya, S. Fuada, K. Afifah, and I. G. Purwanda, “Efficient Android Software Development Using MIT App Inventor 2 for Bluetooth-Based Smart Home,” Wireless Pers. Commun., vol. 105, pp. 233-256, 2019, doi: https://doi.org/10.1007/s11277-018-6110-x
H. Cao, D. G. Cooper, M. K. Keutmann, R. C. Gur, A. Nenkova, and R. Verma, “CREMA-D: Crowd-sourced Emotional Multimodal Actors Dataset,” IEEE Trans. Affect. Comput., vol. 5, no. 4, pp. 377-390, 2014, doi: https://doi.org/10.1109/taffc.2014.2336244
A. Mishra, D. Patil, N. Karkhanis, V. Gaikar, and K. Wani, “Real time emotion detection from speech using Raspberry Pi 3,” in 2017 International Conference on Wireless Communications, Signal Processing and Networking (WiSPNET), Chennai, India, 2017, pp. 2300-2303, doi: https://doi.org/10.1109/WiSPNET.2017.8300170
H. Alshamsi, V. Kepuska, H. Alshamsi, and H. Meng, “Automated Speech Emotion Recognition on Smart Phones,” in 2018 9th IEEE Annual Ubiquitous Computing, Electronics and Mobile Communication Conference (UEMCON), New York, NY, USA, 2018, pp. 44-50, doi: https://doi.org/10.1109/UEMCON.2018.8796594
S. Chebbi and S. Ben Jebara, “On the Selection of Relevant Features for Fear Emotion Detection from Speech,” in 2018 9th International Symposium on Signal, Image, Video and Communications (ISIVC), Rabat, Morocco, 2018, pp. 82-86, doi: https://doi.org/10.1109/ISIVC.2018.8709233
C. Clavel, I. Vasilescu, L. Devillers, G. Richard, and T. Ehrette, “Fear-type emotion recognition for future audio-based surveillance systems,” Speech Commun., vol. 50, no. 6, pp. 487-503, 2008, doi: https://doi.org/10.1016/j.specom.2008.03.012
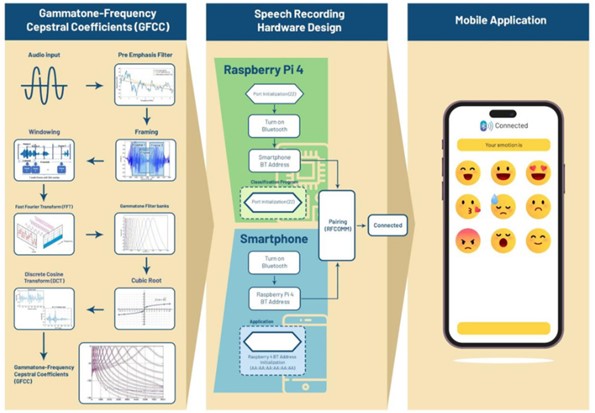
Descargas
Publicado
Cómo citar
Número
Sección
Licencia
Derechos de autor 2024 Revista Mexicana de Ingenieria Biomedica

Esta obra está bajo una licencia internacional Creative Commons Atribución-NoComercial 4.0.
Una vez que el artículo es aceptado para su publicación en la RMIB, se les solicitará al autor principal o de correspondencia que revisen y firman las cartas de cesión de derechos correspondientes para llevar a cabo la autorización para la publicación del artículo. En dicho documento se autoriza a la RMIB a publicar, en cualquier medio sin limitaciones y sin ningún costo. Los autores pueden reutilizar partes del artículo en otros documentos y reproducir parte o la totalidad para su uso personal siempre que se haga referencia bibliográfica al RMIB. No obstante, todo tipo de publicación fuera de las publicaciones académicas del autor correspondiente o para otro tipo de trabajos derivados y publicados necesitaran de un permiso escrito de la RMIB.