Una Mejora del Alineamiento Múltiple de Secuencias con Algoritmos Genéticos: Un Enfoque de Bioinformática en la Ingeniería Biomédica
DOI:
https://doi.org/10.17488/RMIB.45.2.4Palabras clave:
bioinformática, algoritmo genético, alineamiento múltiple de secuencias, msaResumen
El objetivo del presente trabajo es desarrollar una estrategia de procesamiento de información genética para el problema del alineamiento múltiple de secuencias en el área de bioinformática con el propósito de explotar la potencia del hardware y analizar los resultados en términos de calidad de las soluciones obtenidas, así como el tiempo requerido por el sistema de cómputo para realizar el proceso y la determinación del número de funciones evaluadas. Los procedimientos y metodología para dicho trabajo fueron basados en la programación de un Algoritmo Genético en lenguaje Java, del que sucesivamente se obtuvieron cuatro diferentes versiones denominadas Gp1, Gp2, Gp3 y Gp4. Con esto se procesó un conjunto de secuencias genéticas y se evaluaron los resultados a través de la determinación de los perfiles de comportamiento numérico. Entre los hallazgos se encuentra que la diversidad en la población y el procesamiento paralelo tienen influencia en los resultados. A partir del perfil de comportamiento numérico se observa una reducción en el tiempo requerido para realizar el proceso. Se concluye que a) el equipo de cómputo convencional puede generar muy buenos resultados al procesar información genética cuando los algoritmos son preparados para aprovechar al máximo los recursos de hardware. Esto puede guiar al desarrollo de estrategias más eficientes de procesamiento de información genética.
Descargas
Citas
N. Mićić and B. Mićić, “God is Dead, Long Live DNA,” Agro-knowledge J., vol. 18, no. 2, pp. 143-146, Dec. 2017, doi: https://doi.org/10.7251/AGREN1702143M
D. Ghoshdastidar and M. Bansal, “Dynamics of physiologically relevant noncanonical DNA structures: an overview from experimental and theoretical studies,” Brief Funct. Genomics, vol. 18, no. 3, pp. 192–204, Jun. 2018, doi: https://doi.org/10.1093/bfgp/ely026
E. Arunan, “One Hundred Years After the Latimer and Rodebush Paper, Hydrogen Bonding Remains an Elephant!,” J. Indian Inst. Sci., vol. 100, no. 1, pp. 249–255, Jan. 2020, doi: https://doi.org/10.1007/s41745-019-00154-4
A. M. Fleming and C. J. Burrows, “Formation and processing of DNA damage substrates for the hNEIL enzymes,” Free Radic. Biol. Med., vol. 107, pp. 35–52, Jun. 2017, doi: https://doi.org/10.1016/j.freeradbiomed.2016.11.030
É. Leroux, C. Brosseau, B. Angers, A. Angers, and S. Breton, “Méthylation de l’ADN mitochondrial,” Med. Sci., vol. 37, no. 3, pp. 258–264, Mar. 2021, doi: https://doi.org/10.1051/medsci/2021011
E. W. Sayers, E. E. Bolton, J. R. Brister, K. Canese, et al., ‘Database resources of the national center for biotechnology information’, Nucleic Acids Res., vol. 50, no. D1, pp. D20–D26, Jan. 2022, doi: https://doi.org/10.1093/nar/gkab1112
GenBank and WGS Statistics, GenBank, 2024. [Online]. Available: https://www.ncbi.nlm.nih.gov/genbank/statistics/
M. Abdel-Basset, L. Abdel-Fatah, and A. K. Sangaiah, “Metaheuristic Algorithms: A Comprehensive Review,” in Computational Intelligence for Multimedia Big Data on the Cloud with Engineering Applications, A. K. Sangaiah, M. Sheng, Z. Zhang, Eds., Catalunya, Spain: Academic Press, 2018, pp. 185–231. doi: https://doi.org/10.1016/B978-0-12-813314-9.00010-4
S. M. Almufti, A. Ahmad Shaban, Z. Arif Ali, R. Ismael Ali, and J. A. Dela Fuente, “Overview of Metaheuristic Algorithms,” PGSRT, vol. 2, no. 2, pp. 10–32, Apr. 2023, doi: https://doi.org/10.58429/pgjsrt.v2n2a144
D. Rodriguez, D. Gomez, D. Alvarez, and S. Rivera, “A Review of Parallel Heterogeneous Computing Algorithms in Power Systems,” Algorithms, vol. 14, no. 10, art. no.. 275, Sep. 2021, doi: https://doi.org/10.3390/a14100275
X. Wang and J. Liu, “Multiscale Parallel Algorithm for Early Detection of Tomato Gray Mold in a Complex Natural Environment,” Front. Plant. Sci., vol. 12, art. no. 620273, May 2021, doi: https://doi.org/10.3389/fpls.2021.620273
X. Dong, Y. Wu, Z. Wang, L. Dhulipala, Y. Gu, and Y. Sun, “High-Performance and Flexible Parallel Algorithms for Semisort and Related Problems,” in Proceedings of the 35th ACM Symposium on Parallelism in Algorithms and Architectures, New York, NY, USA, 2023, pp. 341–353. doi: https://doi.org/10.1145/3558481.3591071
M. Kimiaei, A. Hassan Ibrahim, and S. Ghaderi, “A subspace inertial method for derivative-free nonlinear monotone equations,” Optimization, pp. 1–28, Sep. 2023, doi: https://doi.org/10.1080/02331934.2023.2252849
A. H. Kamali, E. Giannoulatou, T. Y. Chen, M. A. Charleston, A. L. McEwan, and J. W. K. Ho, “How to test bioinformatics software?,” Biophys Rev., vol. 7, no. 3, pp. 343–352, Sep. 2015, doi: https://doi.org/10.1007/s12551-015-0177-3
Y. Zhang, Q. Zhang, J. Zhou, and Q. Zou, “A survey on the algorithm and development of multiple sequence alignment,” Brief Bioinform., vol. 23, no. 3, art. no. bbac069, May 2022, doi: https://doi.org/10.1093/bib/bbac069
M. Maiolo, X. Zhang, M. Gil, and M. Anisimova, “Progressive multiple sequence alignment with indel evolution,” BMC Bioinformatics, vol. 19, no. 1, art. no. 331, Dec. 2018, doi: https://doi.org/10.1186/s12859-018-2357-1
B. Schmidt and A. Hildebrandt, “Dedicated Bioinformatics Analysis Hardware,” in Encyclopedia of Bioinformatics and Computational Biology, Sydney, Australia: Elsevier, 2019, pp. 1142–1150. doi: https://doi.org/10.1016/B978-0-12-809633-8.20186-6
R. Guo, Y. Zhao, Q. Zou, X. Fang, and S. Peng, “Bioinformatics applications on Apache Spark,” Gigascience, vol. 7, no. 8, art. no. giy098, Aug. 2018, doi: https://doi.org/10.1093/gigascience/giy098
N. A. Stover and A. R. O. Cavalcanti, “Using NCBI BLAST,” Curr. Protoc. Essent. Lab. Tech, vol. 14, no. 1, May 2017, doi: https://doi.org/10.1002/cpet.8
S. Iantorno, K. Gori, N. Goldman, M. Gil, and C. Dessimoz, “Who Watches the Watchmen? An Appraisal of Benchmarks for Multiple Sequence Alignment,” Methods Mol. Biol., vol. 1079, 2014, pp. 59–73. doi: https://doi.org/10.1007/978-1-62703-646-7_4
A. Löytynoja, “Alignment Methods: Strategies, Challenges, Benchmarking, and Comparative Overview,” Methods Mol. Biol., vol. 855, 2012, pp. 203–235. doi: https://doi.org/10.1007/978-1-61779-582-4_7
Y. He, “Research on global double sequence alignment optimization algorithm based on dynamic programming,” in Third International Conference on Computer Science and Communication Technology (ICCSCT 2022), Beijing, China, 2022, art. no. 125060L, doi: https://doi.org/10.1117/12.2662630
J.-H. Hung and Z. Weng, “Sequence Alignment and Homology Search with BLAST and ClustalW,” Cold Spring Harb. Protoc., vol. 2016, no. 11, art. no. pdb.prot093088, Nov. 2016, doi: https://doi.org/10.1101/pdb.prot093088
Q. Zou, X. Shan, and Y. Jiang, “A Novel Center Star Multiple Sequence Alignment Algorithm Based on Affine Gap Penalty and K-Band,” Phys. Procedia, vol. 33, pp. 322–327, 2012, doi: https://doi.org/10.1016/j.phpro.2012.05.069
F. Tang, J. Chao, Y. Wei, F. Yang, Y. Zhai, L. Xu, Q. Zou, “HAlign 3: Fast Multiple Alignment of Ultra-Large Numbers of Similar DNA/RNA Sequences,” Mol. Biol. Evol., vol. 39, no. 8, Aug. 2022, doi: https://doi.org/10.1093/molbev/msac166
B. Reddy and R. Fields, “Multiple Sequence Alignment Algorithms in Bioinformatics,” in Smart Trends in Computing and Communications. Lecture Notes in Networks and Systems, 2022, pp. 89–98. doi: https://doi.org/10.1007/978-981-16-4016-2_9
D. Pacheco Bautista, M. González Pérez, and I. Algredo Badillo, “From sequencing to hardware acceleration of DNA alignment software: A integral review,” Rev. Mex. Ing. Biomed., vol. 36, no. 3, pp. 257–275, Sep. 2015, doi: https://doi.org/10.17488/RMIB.36.3.6
D. Pacheco-Bautista, “ABPSE: Alineador de ADN Basado en Paralelismo a Nivel de Bit y la Estrategia Siembra y Extiende,” Rev. Mex. Ing. Biomed., vol. 40, no. 1, pp. 1–13, 2019. doi: https://doi.org/10.17488/RMIB.40.1.4
A. S. M. Aljohani, F. A. Alhumaydhi, A. Rauf, E. M. Hamad, and U. Rashid, “In Vivo Anti-Inflammatory, Analgesic, Sedative, Muscle Relaxant Activities and Molecular Docking Analysis of Phytochemicals from Euphorbia pulcherrima,” Evid. Based Complement. Alternat. Med., vol. 2022, art. no. 7495867, Apr. 2022, doi: https://doi.org/10.1155/2022/7495867
J. H. Holland, Adaptation in Natural and Artificial Systems. The MIT Press, 1992, doi: https://doi.org/10.7551/mitpress/1090.001.0001
K. Hao, J. Zhao, K. Yu, C. Li, and C. Wang, “Path Planning of Mobile Robots Based on a Multi-Population Migration Genetic Algorithm,” Sensors, vol. 20, no. 20, art. no. 5873, Oct. 2020, doi: https://doi.org/10.3390/s20205873
H. Ahrabian, M. Ganjtabesh, A. N. Dalini, and Z. R. M. Kashani, “Genetic algorithm solution for partial digest problem,” Int. J. Bioinform. Res. Appl., vol. 9, no. 6, pp. 584-594, 2013, doi: https://doi.org/10.1504/ijbra.2013.056622
M. Fernandez, J. Caballero, L. Fernandez, and A. Sarai, “Genetic algorithm optimization in drug design QSAR: Bayesian-regularized genetic neural networks (BRGNN) and genetic algorithm-optimized support vectors machines (GA-SVM),” Mol. Divers, vol. 15, no. 1, pp. 269–289, Feb. 2011, doi: https://doi.org/10.1007/s11030-010-9234-9
M. Sale and E. A. Sherer, “A genetic algorithm based global search strategy for population pharmacokinetic/pharmacodynamic model selection,” Br. J. Clin. Pharmacol., vol. 79, no. 1, pp. 28–39, Jan. 2015, doi: https://doi.org/10.1111/bcp.12179
S. H. Almanza-Ruiz, A. Chavoya, and H. A. Duran-Limon, “Parallel protein multiple sequence alignment approaches: a systematic literature review,” J. Supercomput., vol. 79, no. 2, pp. 1201–1234, Feb. 2023, doi: https://doi.org/10.1007/s11227-022-04697-9
D. Song, J. Chen, G. Chen, N. Li, et al., “Parameterized BLOSUM Matrices for Protein Alignment,” IEEE/ACM Trans. Comput. Biol. Bioinform., vol. 12, no. 3, pp. 686–694, May 2015, doi: https://doi.org/10.1109/tcbb.2014.2366126
J. S. Piña, S. Orozco-Arias, N. Tobón-Orozco, L. Camargo-Forero, R. Tabares-Soto, and R. Guyot, “G-SAIP: Graphical Sequence Alignment Through Parallel Programming in the Post-Genomic Era,” Evol. Bioinform. Online, vol. 19, art. no. 117693432211505, Jan. 2023, doi: https://doi.org/10.1177/11769343221150585
I. R. and A. Chavoya, “PaMSA: A Parallel Algorithm for the Global Alignment of Multiple Protein Sequences,” IJACSA, vol. 8, no. 4, pp. 513-522, 2017, doi: https://dx.doi.org/10.14569/IJACSA.2017.080468
T. Harada and E. Alba, “Parallel Genetic Algorithms,” ACM Comput. Surv., vol. 53, no. 4, pp. 1–39, Jul. 2021, doi: https://doi.org/10.1145/3400031
J. Zhu, G. Wang, Y. Li, Z. Duo, and C. Sun, “Optimization of hydrogen liquefaction process based on parallel genetic algorithm,” Int. J. Hydrogen Energy, vol. 47, no. 63, pp. 27038–27048, Jul. 2022, doi: https://doi.org/10.1016/j.ijhydene.2022.06.062
J. Xu, L. Pei, and R. Zhu, “Application of a Genetic Algorithm with Random Crossover and Dynamic Mutation on the Travelling Salesman Problem,” Procedia Comput. Sci., vol. 131, pp. 937–945, 2018, doi: https://doi.org/10.1016/j.procs.2018.04.230
K. R. Anil Kumar and E. R. Dhas, “Opposition based genetic optimization algorithm with Cauchy mutation for job shop scheduling problem,” Mater. Today Proc., vol. 72, pp. 3006–3011, 2023, doi: https://doi.org/10.1016/j.matpr.2022.08.263
T. D. Pham and W.-K. Hong, “Genetic algorithm using probabilistic-based natural selections and dynamic mutation ranges in optimizing precast beams,” Comput. Struct., vol. 258, art. no. 106681, Jan. 2022, doi: https://doi.org/10.1016/j.compstruc.2021.106681
Galaxy Community, “The Galaxy platform for accessible, reproducible and collaborative biomedical analyses: 2022 update,” Nucleic Acids Res., vol. 50, no. W1, pp. W345–W351, Jul. 2022, doi: https://doi.org/10.1093/nar/gkac247
A. Bonilla-Petriciolet, J. C. Tapia-Picazo, C. Soto-Becerra, and J. G. Zapiain-Salinas, “Perfiles de comportamiento numérico de los métodos estocásticos simulated annealing y very fast simulated annealing en cálculos termodinámicos,” Ing. Inv. Tecnolog., vol. 12, no. 1, pp. 51–62, Jan. 2011, doi: https://doi.org/10.22201/fi.25940732e.2011.12n1.006
R.-W. Ernesto, L.-G. Ernesto, B. Rafael, and G.-G. Yolanda, “Perfiles de comportamiento numérico de los métodos de búsqueda immune network algorithm y bacterial foraging optimization algorithm en funciones benchmark,” Ing. Inv. Tecnolog., vol. 17, no. 4, pp. 479–490, Oct. 2016, doi: https://doi.org/10.1016/j.riit.2016.11.007
E. D. Dolan and J. J. Moré, “Benchmarking optimization software with performance profiles,” Math. Program., vol. 91, no. 2, pp. 201–213, Jan. 2002, doi: https://doi.org/10.1007/s101070100263
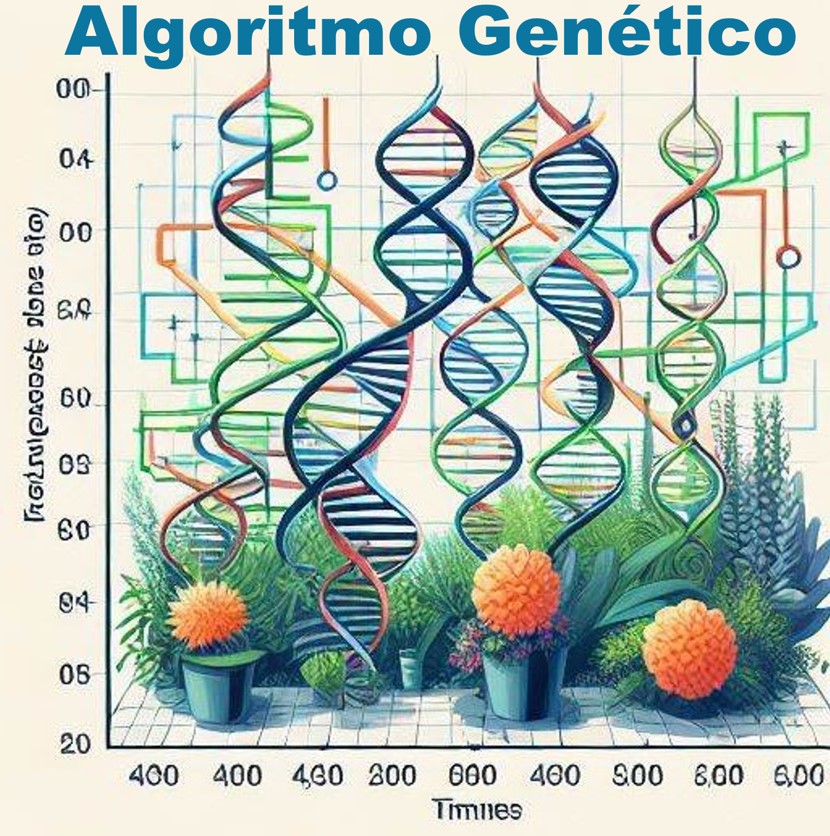
Descargas
Publicado
Cómo citar
Número
Sección
Licencia
Derechos de autor 2024 Revista Mexicana de Ingenieria Biomedica

Esta obra está bajo una licencia internacional Creative Commons Atribución-NoComercial 4.0.
Una vez que el artículo es aceptado para su publicación en la RMIB, se les solicitará al autor principal o de correspondencia que revisen y firman las cartas de cesión de derechos correspondientes para llevar a cabo la autorización para la publicación del artículo. En dicho documento se autoriza a la RMIB a publicar, en cualquier medio sin limitaciones y sin ningún costo. Los autores pueden reutilizar partes del artículo en otros documentos y reproducir parte o la totalidad para su uso personal siempre que se haga referencia bibliográfica al RMIB. No obstante, todo tipo de publicación fuera de las publicaciones académicas del autor correspondiente o para otro tipo de trabajos derivados y publicados necesitaran de un permiso escrito de la RMIB.